Over the past two months we’ve been discussing
predictive analytics in detail, which has generated a lot of interest from readers. Building on that start, we’re now moving to another series on a related topic – Real-time data visualization.
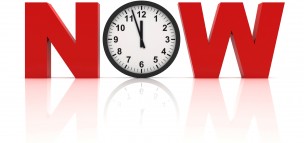
‘Real-time’ has become a buzzword in recent times. Every other product we come across touts its real-time capabilities. When evaluating a new tool for your marketing, sales, or operational needs, you may have considered real-time as a necessary requirement. When reading articles on popular tech blogs, you’ve probably come across the term every other day. Yet, for many, the phrase ‘real-time’ is like a popular stranger that everyone talks about, but a stranger nonetheless. They wish they knew what all the buzz is about, but don’t know where to start. If that’s you, this series will serve as a great starting point in your understanding of what real-time data is all about.
In this series of blog posts, we discuss how businesses are approaching real-time business intelligence in a way that empowers their decisions. We’ll take a look at the various components of a real-time business intelligence system, with an emphasis on the data visualization aspect.
We begin by assessing where we are today, the role big data plays in the functioning of our businesses, and how we got here.
The Three V’s of Big Data
With the explosive growth of data creation in recent years, and the opportunities afforded by big data, the need for real-time business intelligence is growing by the day. IBM estimates that 90% of all the world’s data has been created in the past two years alone. However, while this firehose of data hits us at ever increasing speeds, IDC observes that less than 1% of all available data is analyzed. Businesses face the problem of data, data everywhere, not a second to think.
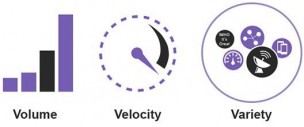
About a decade ago, Gartner ushered in a new era of business intelligence when they drew a contrast between data as we knew it back then, and the way data was headed. They suggested three prominent characteristics of this new type of data called big data – volume, velocity, and variety. Since then, many have tried to add many more V’s like value, veracity, and viability. We’ve also seen some backlash against this
‘wanna V’ syndrome. For our purposes, we’ll stick to the original definition.
When discussing real-time analytics, while none of the three V’s can be left out, velocity is of prime importance. This is because of the speed with which data is captured, stored, processed, and visualized. This velocity is what differentiates real-time analytics from traditional BI systems. The entire cycle from data capture to final action taken on it can happen in a matter of seconds, or even milliseconds.
From Relational Databases to Big Data
Real-time analytics wasn’t possible a decade back. It was enough for a store owner to know how many items of a product were sold each day, and which were the fastest selling. This information aggregated over a quarter would assist with making strategic decisions, for example, about which product lines demonstrated the most growth potential. Businesses were happy to store data in relational databases, which accepted data only in a pre-defined structure. The storage and processing was heavily restricted by the ever-increasing number of physical servers. The stored data was queried weekly or monthly using SQL queries that were processed in a sequential order, making it impossible to run complex, large-scale queries. Also, these queries would return results successfully only if all databases involved were functional, and the data was stored in a consistent manner. This was never going to hold up in a world that was about to get much more complex with the advent of e-commerce, web analytics, social media, and mobile devices.
Fast forward to today, and the same store owner is not satisfied with getting just the history of last quarter’s sales. On an operational level, they want a real-time BI system that knows what a particular customer purchased just now, what they’re most likely to buy next, and which add-ons to suggest right now, before the customer’s attention fades. This sort of real-time, predictive analytics wasn’t possible back in the days of traditional data warehousing. But today, with vastly reduced storage prices, increased RAM capabilities, powerful querying tools, and capable visualization components, real-time analytics is becoming a reality in more and more business scenarios. It is now not only possible, but imperative that enterprises master the art of real-time business intelligence for both strategic and tactical purposes.
Below is a video that illustrates the impact of big data and real-time analytics on retailers. We’ll, of course, be talking beyond just the retail industry, but this example from retail is a great starting point.
With that we conclude our introduction to this series, and will pick it up from here next week. In the upcoming posts, we’ll go behind the scenes to understand what goes into the making of a real-time business intelligence system. We’ll then look at some applications of real-time analytics, and scan the technological landscape to understand what makes these innovations possible. Finally, we’ll put together a broad list of requirements that you can keep handy when choosing a real-time data visualization component. If any aspect of your product or business depends on real-time data, this series is a 101 in real-time analytics that you can’t afford to miss.
If you can’t wait for the rest of the posts, you can always get our free white paper titled ‘
The Ultimate Guide to Real-time Data Visualization’ which covers this topic in great detail. Of course, in this blog post series we’ll be including lots of practical examples, extending the thought in the white paper. So, you may want to go through the white paper to get an overview of the topic, but do stay tuned to the blog posts for in-depth treatment of each section of the white paper.